Geospatial Analytics
Machine Learning & Data Science
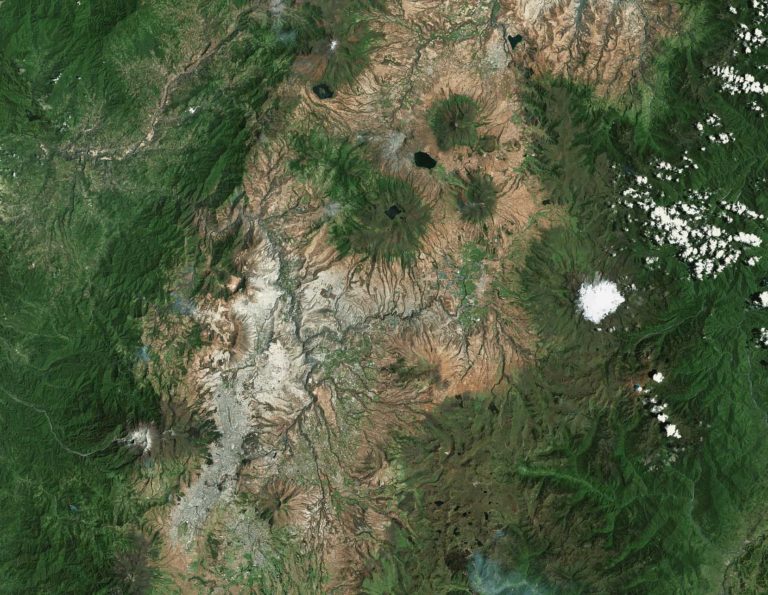
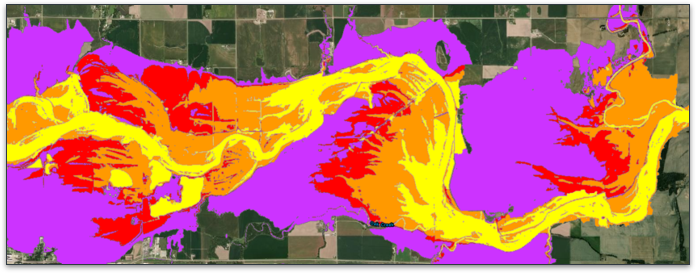
Advanced geospatial analytics driven by machine learning and other AI techniques requires a vision to achieve. Your vision isn’t cookie cutter, so your AI application shouldn’t be either. With Lynker Intel’s guidance, you can integrate advanced analytics and data science into your strategy – and understand the strengths and weaknesses of various methods based on your goals. Lynker Intel brings open-source solutions to our clients with well-researched and well-documented approaches to develop customized analytical solutions to problems like flood forecasting, natural hazards and risk assessment, and wetland delineation.
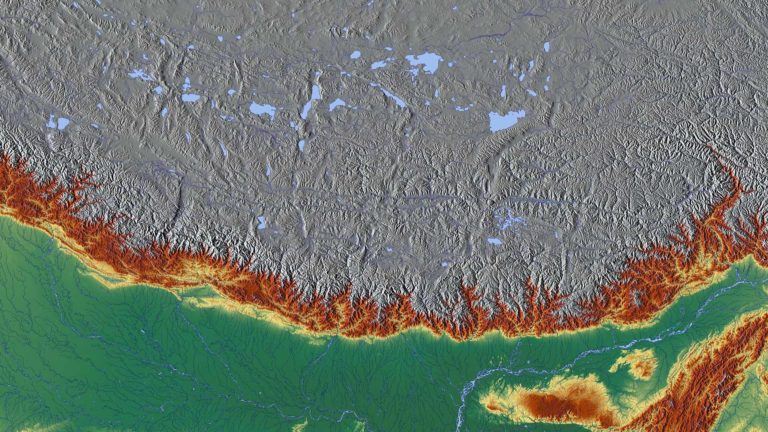
Lynker Intel’s smart maps pinpoint areas of potential risk, reveal deeper insights on how to manage risk, and provide a common view that streamlines communication during all phases of risk assessment and management. We can help you prioritize where to allocate resources with data-driven risk assessment and by using our predictive analytics to reveal patterns and trends. Lynker scientists use spatial analysis, data science, and location as the connective thread to uncover hidden patterns and trends for enhanced risk assessment. Our competitive edge comes from our ability to integrate location into Lynker Analytics machine learning models applied to big data and Internet of Things (IoT) technology to make quick, informed decisions based on the most up-to-date information.
Lynker Intel develops actionable spatial intelligence for decision makers – uncertainties are clearly communicated and valued, and we develop the customized tools needed to support decision-making under uncertainty. The use of platforms and online tools can provide accessible, credible and transparent information on past and future climate behavior. Lynker Intel can work with clients to ensure that our actionable intelligence is complemented with the development of the technical and institutional capacity to manage climate-related and other risks.
Lynker Intel tools for mainstreaming adaptation in critical policy areas and encouraging investments in resilient infrastructure include:
- Spatial representations of natural hazards and risk, including multi-hazard and risk assessment GIS;
- Spatial planning frameworks to better inform maximum probable loss (MPL) models to compute insurance premiums and to redirect development away from high-risk areas;
- infrastructure project and policy appraisals, including Strategic Environmental Assessment and Environmental Impact Assessment; and
- regulatory and economic standards (such as building codes).
Relevant Case Study
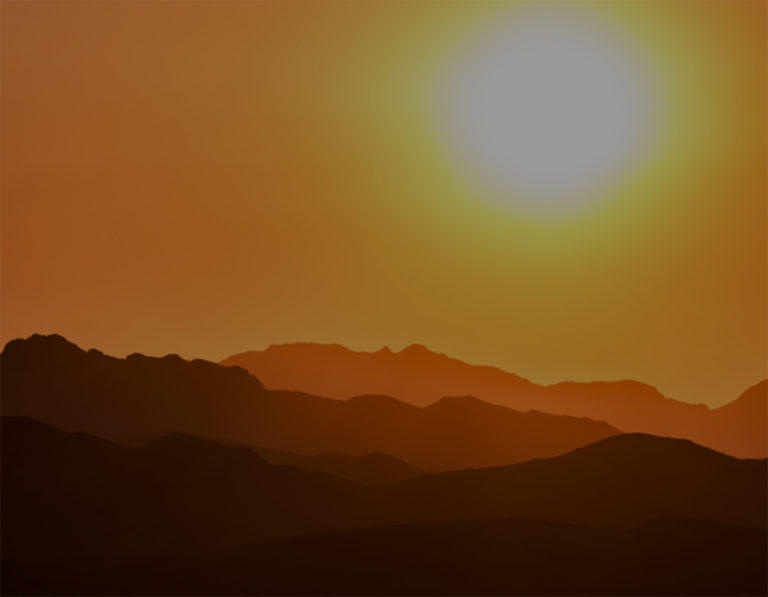
Future Avoided Cost Explorer: Hazards
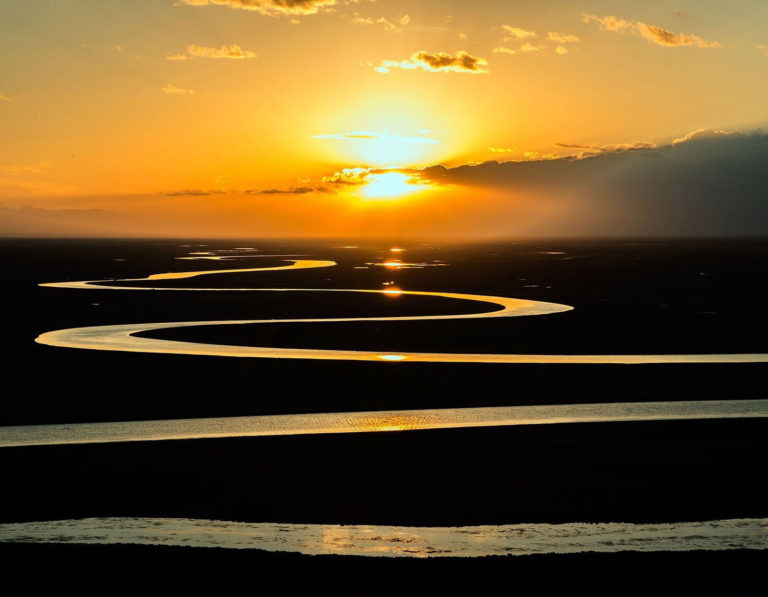